When consumers are harmed by defective products or pharmaceuticals, they often seek legal counsel to pursue liability claims. These cases are complex, and law firms need cutting-edge solutions to manage them and assess their chances of successfully representing these consumers.
A company offering innovative solutions for case management wanted to build a machine-learning model that predicts the outcome of cases in the litigation space and enhances decision-making. However, they struggled with a few challenges:
- Limited internal resources
- Insufficient stakeholder support
- The inability to transform concepts into production-ready ML models, largely due to cost concerns
Recognizing these challenges, the client sought external support to help them transition from foundational analytics to achieving more advanced, impactful outcomes with machine learning.
BlueCloud’s adaptable, cost-effective Borderless Delivery Model helped them navigate these challenges, achieving what many companies fail to do—bringing machine learning models into production, defying industry norms where almost 90% of such projects never reach that stage.
In this article, we will walk you through the steps BlueCloud took to develop two machine-learning models to predict case outcomes in the litigation space. We will also demonstrate how our flexible Borderless Delivery Model enables the rapid creation of sustainable, ML-powered case management solutions that help companies assess and enhance their chances of success.
Build or Buy an ML Model? Breaking Down the Best Approach
The client faced one of the most common dilemmas—whether to build or buy an ML model. They considered opting for an ML tool, which, while capable of delivering results, would be an AI black-box solution, lacking transparency and dependent on external vendors. On the other hand, building an in-house team was another big challenge due to the prohibitive cost of data science talent.
Ultimately, they decided to build the model with BlueCloud’s assistance, leveraging their expertise to create a transparent, effective solution that balanced cost and functionality.
Moving from Foundational Analytics to Advanced Analytics Solution
BlueCloud worked on an initial analytics project that provided a foundation for the client, helping them see the strategic value, build credibility with their current and prospective clients, and justify their further investment.
As a trusted partner, BlueCloud focused on helping the client truly understand the why behind employing ML models to ensure they advance from use cases to new performance frontiers.
BlueCloud's role was pivotal in helping the client move from basic analytics to advanced predictive outcomes.
The team started with a pilot project to demonstrate its ability to build an effective predictive model and showcase outputs that would lay the foundation for future production phases. This process typically involves extensive time and resources, but BlueCloud’s expertise and deep knowledge of ML accelerated these stages, delivering results in just a few weeks.
Additionally, the BlueCloud team conducted a cost-benefit analysis to determine whether Snowflake or AWS would be more suitable for the client’s machine learning needs, ultimately guiding them towards Snowflake for cost-effectiveness and functionality.
From Endless Silos to Unified Data with Snowflake
BlueCloud’s dedication to Snowflake workloads and deep expertise in the Snowflake Platform and Data Cloud played a critical part in enabling the client to extract valuable insights for advanced case management, enhanced decision-making, and better outcomes in the litigation space.
Snowflake, a platform that powers the AI Data Cloud, is designed to help thousands of organizations mobilize their data to the cloud with near-unlimited scale, concurrency, and performance.
Predicting Wins with ML and MLOps: How Did We Do It?
From process automation to customer experience personalization, ML-powered solutions push the boundaries of what can be achieved across industries and organizations worldwide. One standout use case of machine learning is its ability to extract valuable insights from diverse datasets that drive predictive analytics, transform decision-making, and improve outcomes.
So, how did we help the client achieve their goal?
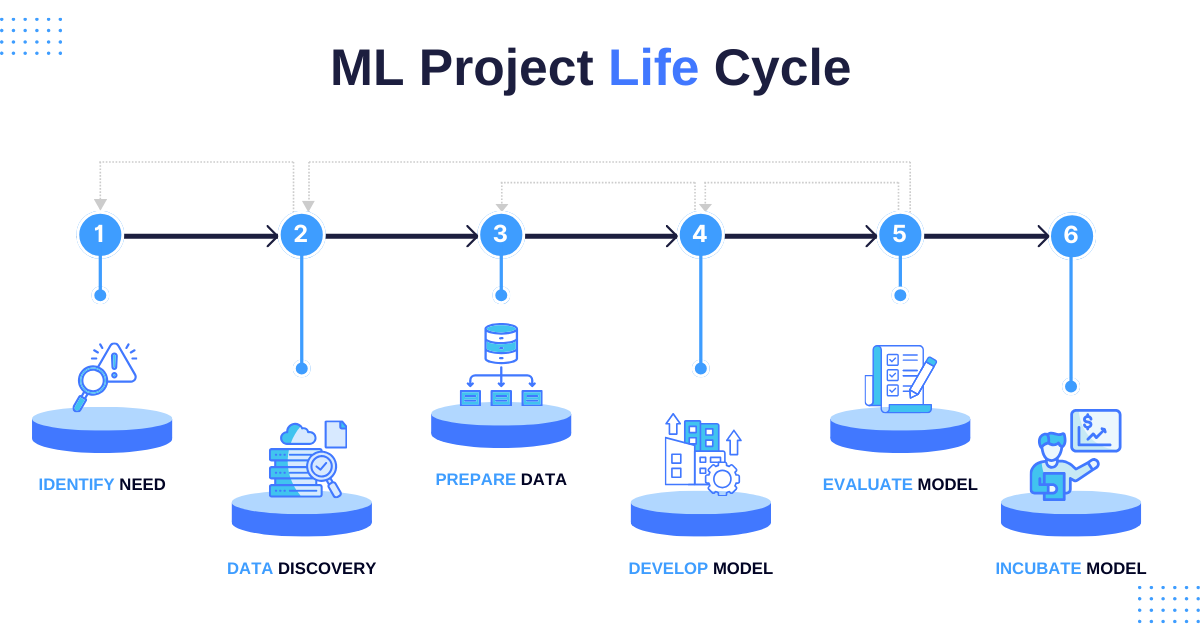
MLOps holds the key to unlocking the enormous potential of AI. BlueCloud’s machine learning consulting services are built on deep domain expertise and a strong history of successful ML implementations.
We emphasize clear communication and a collaborative, problem-solving approach to deliver optimal outcomes for our clients. We help companies build solutions that enable them to make predictions, automate decision-making, create innovative products and services, and improve their bottom line fast.

Introducing ML Models
To predict case outcomes and prioritize those more likely to have positive outcomes, BlueCloud developed two machine learning models, pre-intake model and post-intake model.
These models use historical data to identify patterns between key features and predict case results based on those patterns.
The pre-intake model plays a crucial role in the early stages of case processing. After cases are ingested into the system, either through an API or manually, initial data become available. Before proceeding with further steps, the pre-intake model uses this initial data to predict the outcome, streamlining the process from the start.
The post-intake model is activated after the intake process is completed. It can predict the potential outcome at this stage, which helps efficiently manage cases through subsequent steps like document requests, gathering, QC, and reviews, all of which require significant effort. Efficient distribution of resources is crucial, and the data model supports this process.
From Pilot to Production
Building machine learning models requires a lot of components and moving parts. Our team of ML professionals took a business value focused approach to deliver on the initial use case, coupled with a MLOps mindset to help operationalize the development, deployment and maintenance of the machine learning models in production. BlueCloud helped to navigate all the complexities and deliver the two ML models and a scalable framework for expansion in a matter of weeks.
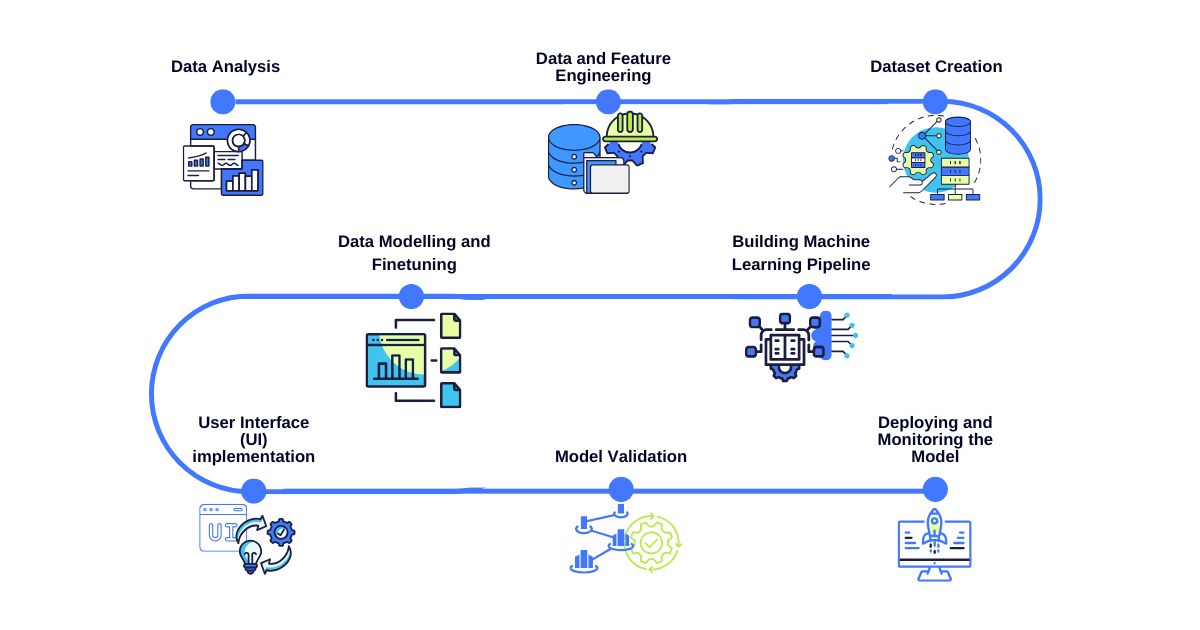
What Are the Key Differences between Pre-Intake and Post-Intake Model?
Here are the key differences between the post-intake and pre-intake pipelines:
- Feature Utilization: The post-intake model includes the feature that tracks the state of the case after intake, unlike the pre-intake model where this feature is excluded due to incomplete intake states. The post-intake model also incorporates features like the number of states passed and counts of events and documents from previous states.
- Scoring and Data Tables: The post-intake scores tables indicate the state at which scores are generated. This allows the post-intake model to produce different scores for each state of a case, unlike the pre-intake model which generates a single score per case.
- Data Splitting: The pre-intake model uses a random train-test split, while the post-intake model employs a case-based split to avoid having the same cases in both training and test sets. This approach ensures more reliable performance evaluation and allows flexibility in the splitting method.
Achieving Rapid Sustainable Business Success with BlueCloud’s Flexible Borderless Delivery Model
The rapid delivery of the pilot within weeks provided the client with the momentum to proceed to the following phases.
BlueCloud’s flexible approach—built on the Borderless Delivery Model, allowed the team to not only deliver the solution but also transfer knowledge to the client’s team, ensuring they could maintain and evolve the solution independently. This transfer of expertise was key – it empowered the client to own and manage the solution long-term, turning a short engagement into a sustainable success.
What Do You Want to Achieve with ML?
Millions of businesses use ML for routine decisions, but when applied at scale, it can tackle big strategic questions. By leveraging data across an organization, ML models can predict the impact of major initiatives, like Supply Chain Optimization, reaching zero CO2 or transitioning to electric fleets.
This shifts decision-making from gut instincts to data-driven insights, turning uncertain leaps into calculated, well-informed strategies.
Building an advanced case management predictive model is one such application, with the potential to revolutionize the litigation space, saving significant time and money while safeguarding people’s rights.
Here we offered a compliant approach that prioritizes areas with the highest likelihood of success and the ability to review areas of improvement within cases that had a lower likelihood to improve success rates.
BlueCloud's involvement illustrated how a strategic partner can bridge the gap between initial analytics and production, using innovative Snowflake functionality to deliver impactful, long-lasting solutions. This narrative highlights the practical benefits of bringing in a knowledgeable partner not just to complete a task but to ensure sustainable success.
--
Explore our AI and ML services to learn how we can help you transform insights into actions and make date-driven decisions to fuel your business growth.
Learn more about how Snowflake can help you maximize the value of your data here.